Wildfires are a natural component of the Earth system, important for nutrient release and vegetation growth. Climate change, however, is contributing to more frequent, more destructive and less predictable wildfires worldwide. Australia, in particular, experiences regular bush fires during the summer, but the devastating 2019-2020 bushfire season, known as the Black Summer, was unprecedented in its severity and scale, killing dozens of people and destroying thousands of homes.
Quantifying and monitoring fires is fundamental to mitigate their negative impact on the environment and society, but also for the ongoing climate studies, as wildfires have a significant influence on global atmospheric emissions and climate change. The increasing availability of Earth Observation (EO) data combined with the advanced analytics provided by Artificial Intelligence (AI) and Machine Learning (ML), along with the exceptional processing power of cloud computing, has allowed the AI4EO wildfire project, led by CGI UK, to generate a service that can map fires at an unprecedented level of detail and also provide fast, reliable and accessible information as required by the wildfire fighting community.
AI to assist in burnt area mapping
The application of AI to Burned Area (BA) mapping is an important potential step to enhance current solutions. Fire occurs in different locations across the world, at different intensities and for varying durations. The signal of these fires when seen from satellite data can be significantly different. The figure below shows examples of what fires and burned areas look like using a false colour composite from Sentinel-2.
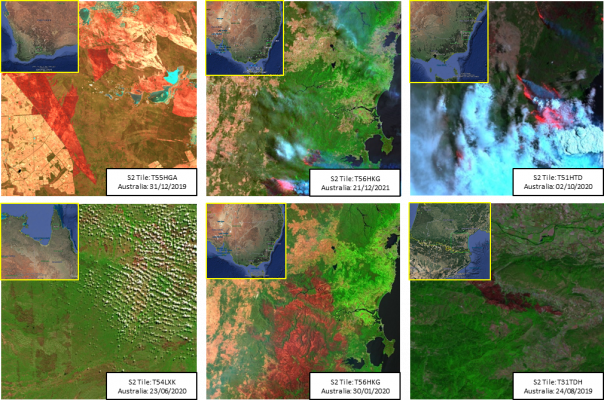
AI offers a solution to deal with these mixed signals, defined by the data used to train the algorithm. In response to this, the selected AI algorithm has been trained using a large reference data set generated by the University of Leicester as part of ESA’s Climate Change Initiative (CCI), which provides unprecedented spatial and temporal distribution that characterise the nature of vegetation fires globally. The accuracy of the algorithm was tested on thirteen reference sites across Australia and France obtaining an average accuracy score of 81%.
An Australian example: the Upper Murray fire
The AI4EO wildfire team has used examples of different fire events across Australia to assess the quality and accuracy of the developed algorithm against data provided by Australia’s wildfire management organisations. One example is the Upper Murray – Victoria fire, where nearly ¼ of a million hectares burned for several months from December 2019 to February 2020. This was an extremely complex fire that consumed many different types of vegetation, and run across two states in Australia. The evolution of this fire is summarised in the maps below (Figure 2). The AI4EO approach mapped 85% of the reference burned area. Throughout the country, the developed BA mapping products have consistently maintained a high level of accuracy when compared to the manually measured reference data provided.
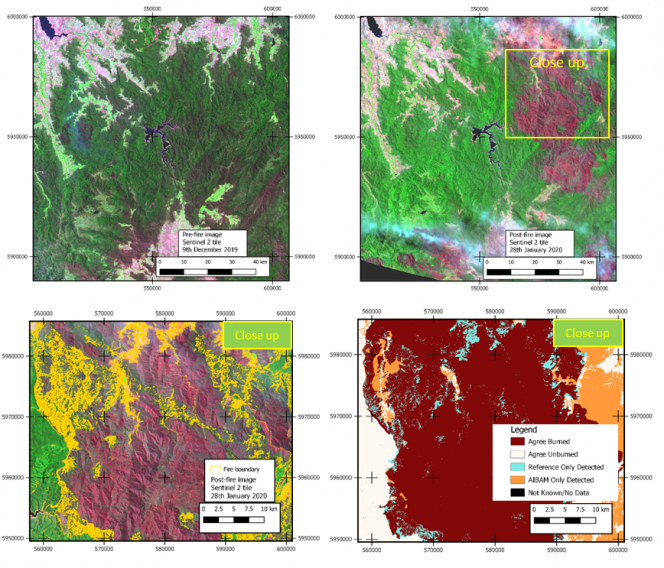
An open, accessible demonstration service
The developed algorithm has been made available to the wildfire management and environmental community as an Artificial Intelligence Burned Area Mapping (AIBAM) product. The AIBAM service can be accessed through the EO4SD Lab (to request your free EO4SD Lab account please contact us directly at support@eo4sd-lab.net), an online portal that provides access to an extensive archive of EO data along with tools, workflows and services to derive useful information from such data (Figure 3). A number of demonstration products, covering wildfire events relevant to the users have been generated and shared. Feedback has been positive with users recognising the products can complement and extend their existing capabilities. A selection of these products are available through the EO4SD Lab’s EOWiki interface.
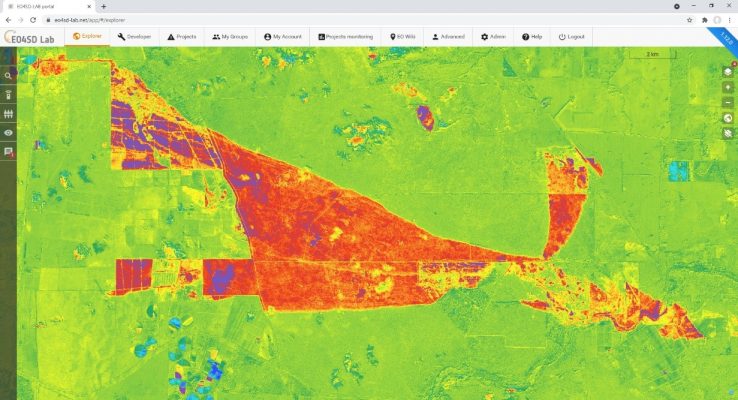
What next
The AI4EO project and demonstration service has shown the potential of combining increasingly frequent and high-resolution satellite observations with Artificial Intelligence using Machine Learning to provide improved Burned Area mapping products to support wildfire management organisations.
Machine Learning enables the service to be easily trained using real wildfire events over a range of differing biomes and scenarios to create a collection of mapping solutions. When executed, the demonstration service automatically selects the most relevant mapping solution to the scene, allowing the creation of a simple, easy to interpret, map of Burned Areas. Deploying the service on a cloud-based online processing platform, such as the EO4SD Lab, provides users with a robust, scalable service that creates Burned Area maps, which can be easily analysed and ingested into the user’s established systems.
The project findings, as discussed in this article, show the current good capabilities of the AIBAM service. The AI4EO project team are currently devising a roadmap, incorporating user feedback, for service improvements to be undertaken in the next phase of the project. These improvements include developing a more robust central classification algorithm, through further Machine Learning training on a wider variety of biomes and fire scenarios as well as exploring additional Machine Learning algorithms, such as Convolutional Neural Network, to create a single universal mapping solution that can be applied on any wildfire. Other improvements focus on the ease of use of the AIBAM service, such as automatic satellite imagery selection and service execution.