Snow cover is an important component of the Earth’s cryosphere and plays a significant role in regulating the Earth’s climate and hydrological cycle. Accurate estimates of snow cover are essential for a range of applications, from climate modelling to water resource management. In a recently published study, researchers develop an adaptive method for estimating snow-covered fraction at various spatial scales using open EO data.
State of the art
Satellite remote sensing has emerged as the most viable option for mapping the largest component of the cryosphere – seasonal snow – due to the extensive area it covers each year (around 50% of the landmass in the Northern Hemisphere). Currently, there is a plethora of snow-cover products available at continental-to-global scales, with most being binary products with medium-to-low ground resolution (ranging from 0.005 to 0.25∘, or about 0.5 to 25 km). These products typically use only a few spectral bands to differentiate between snow-covered and snow-free regions. However, the lack of a quantitative uncertainty estimate for individual pixels is a major drawback of binary snow products.
As for estimating snow-covered fraction (SFC), there are three popular approaches: normalized difference snow index (NDSI), multispectral unmixing, and machine learning.
NDSI effectiveness is hampered by shadows, associated to clouds and topography. On the other hand, multispectral unmixing and machine-learning methods have shown to be more adaptable in terms of data input and have exhibited promising outcomes. However, current significant multispectral unmixing applications employ endmember spectra from spectral libraries, with snow-free and snow-covered signatures that vary greatly both locally and globally, making it difficult to implement the approach for global-scale applications.
The new proposed approach
A new method (Locally Adaptive Multi Spectral Unmixing – LAMSU) for estimating the snow-covered fraction (SCF) from multispectral optical satellite data has been developed in a study funded by ESA Snow CCI and EO Science for Society AlpSnow – Alps Regional Initiative projects. The method is suitable for high-to-medium spatial resolution sensors such as those onboard Sentinel-2, Landsat 8/9, Sentinel-3, Suomi National Polar-orbiting Partnership, and Terra. It is adaptable to local variations in solar illumination and spatial variations in snow-free and snow-covered land surfaces, making it applicable to a wide range of environments. The method also provides a pixel-wise estimate of the SCF uncertainty using error propagation.
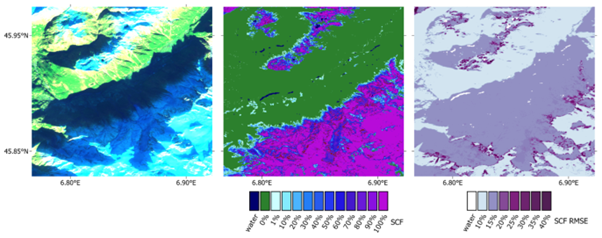
Results obtained from high-resolution sensors (on Sentinel-2, Landsat 8/9) were validated using very-high-resolution data from WorldView-2/3 with random sampling to resolve the unequal distribution of snow-free and snow-covered pixels in the scenes. The consistency of the SCF estimates was also tested through intercomparison of medium-resolution LAMSU SCF estimates from Sentinel-3 SLSTR/OLCI, Suomi NPP VIIRS, and Terra MODIS. The multi-sensor bias and multi-sensor RMSE were used to quantify the consistency between each sensor pair.
The presented methodology, LAMSU, performed consistently for all five different high- and medium-resolution satellite sensors, and it is expected to allow for tractable large-scale multi-sensor processing to estimate the SCF globally in near-real-time with a quantitative uncertainty. The algorithm will be further developed and tested with hyperspectral data from EnMAP and PRISMA missions as a contribution to the scientific preparation for the proposed Copernicus Expansion Mission CHIME. A multi-sensor snow product combining data from sensors that capture the Earth’s surface at different resolutions will also be developed.
Featured image: Snow cover in the Alps on a Copernicus Sentinel-3 image captured on 18 December 2022. Contains modified Copernicus Sentinel data (2022), processed by ESA, CC BY-SA 3.0 IGO
References
Keuris, L.; Hetzenecker, M.; Nagler, T.; Mölg, N.; Schwaizer, G. An Adaptive Method for the Estimation of Snow-Covered Fraction with Error Propagation for Applications from Local to Global Scales. Remote Sens. 2023, 15, 1231. https://doi.org/10.3390/rs15051231