In a world where public health threats are increasingly challenging, wide availability of EO data, increased computational power and AI untapped potential open new opportunities to create early warning systems and support public authorities in decision making.
The AIDEO project explored the use of AI applied to a pool of EO datasets in the context of the West Nile Disease (WND), a widespread zoonosis caused by a vector-borne virus.
First isolated in Uganda in 1937, West Nile Virus was described as the cause of a West Nile fever epidemic in humans in Israel in 1951. The role of mosquitos and birds as virus reservoirs was delineated in the Nile delta a few years later. Since the end of the 90s the disease has been widely reported in the Western Hemisphere, throughout whole America, and in Europe, causing equine and human health threat.
In Italy, since 2016, an integrated approach has been applied with the veterinary and human surveillance activities coordinated in a single national plan (One Health surveillance). While the virus transmission cycle is well understood, with birds acting as the primary hosts and mosquito vectors transmitting the virus to other birds and humans and horses as occasional dead-end hosts, identifying suitable environmental conditions across large areas containing multiple species of potential hosts and vectors can be difficult.
Climatic and environmental factors from EO data: predicting the spread in Italy
Copernicus SSM data together with day/night LST and NDVI data from MODIS have been used to populate a data cube to study infected areas, as registered by the local veterinary authorities into the National Animal Disease Notification System (SIMAN), in the 160 days preceding the reported infection.
EO data – pre-processed to fill gaps (e.g. cloud cover) and resampled at 250 m resolution – and veterinary cases – converted into binary raster maps aggregating the points at 250 m spatial and 16 days temporal resolution – have been used as input to a ML extreme gradient boosting model aimed at answering the following question: considering the climatic and environmental conditions recorded in the past time steps (from time step 1 to time step 10) which is the probability of WNV circulation in the following 16-days’ time step?
The study, fully described in a recently published paper, used 2017-2018-2019 data for training, validating and testing the model and proved to perform quite well (0.84 overall accuracy for the 2019 epidemic test dataset).
The country-wide application of the model detects the difference among the three epidemics, in particular, the different onset of cases in 2018 compared to 2017 and 2019, confirming that the climatic and environmental conditions of the first 5 months of the year may have played a different role in amplifying WNV circulation.
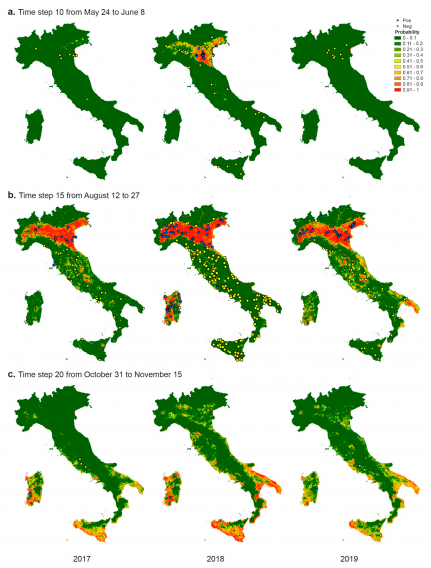
The different lengths of the period favourable for the virus circulation may influence the chance of having the passage of the virus from the enzootic cycle (birds and mosquitoes) to mammals (equids and human beings).
Can AI help see virus circulation thanks to EO data spectral richness?
The objective of improving detection of infected areas is behind another component of the AIDEO project activities, focused on deep learning models to pre-train a dataset before performing the final feature extraction task.
In the study, fully described in a paper, a self-supervised learning approach for multi-band satellite imagery is proposed and applied to Sentinel-2 datasets.
It consists of two steps: firstly, an encoder-decoder architecture is used to predict RGB color channels from those capturing spectral information (colorization); secondly, the encoder is used as a pre-trained feature extractor for a classification task (in this case, the binary identification of positive and negative West Nile Disease sites).
The initialization (colorization) leads to remarkable results, exceeding other common approaches (e.g. ImageNet) especially in presence of scarce labelled data and when used in ensemble with RGB channels.
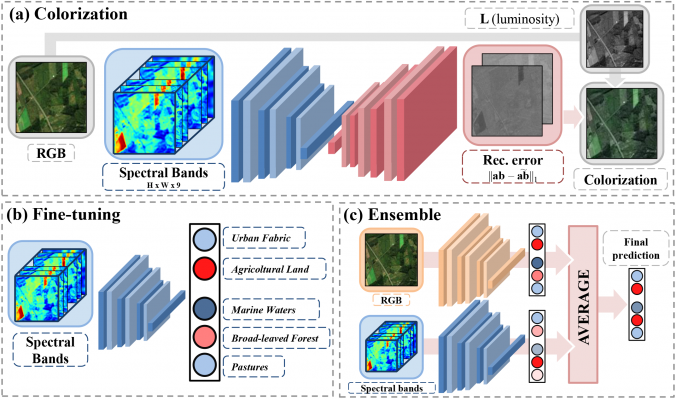
The way forward
Results of AIDEO project activities to date appear very promising for expanding our knowledge and developing operational predictive tools for vector-borne diseases. Using more consistent time series on virus detection in mosquito pools could improve the performance of the prediction model and its applicability, together with the availability of true negatives with the corresponding date of collections, rather than pseudo-absence data.
Setting up a pipeline that makes systematic use of EO data and integrating the model into a solid early warning system would allow a better targeted surveillance and public health interventions in the upcoming West Nile disease seasons.