Incomplete avalanche activity datasets introduce uncertainty to avalanche forecasting
Roughly 150-250 people die each year in avalanche accidents. Infrastructure loss and costs from evacuations and road closures and the use of active and permanent mitigation measures amount to large economic costs.
Regional avalanche forecasting is an active mitigation measure that informs the public of actual and future avalanche risk in regions typically several square-km large. For avalanche forecasting, the knowledge of spatio-temporal avalanche activity is critical, however, difficult to come by. Traditional field-based observations have observer-bias and are bound to small, accessible areas.
Spatio-temporal complete avalanche activity datasets
Norut (officially merged with NORCE as from 1 April 2019) has developed a near-real time processing chain that automatically detects avalanches in Sentinel-1 images and outputs vectorized avalanches within roughly 30 min after data download. The avalanche polygons contain metadata on release timing and location, topographical conditions (slope aspect, angle and elevation) and morphological features (area, length, width). With this processing chain, large regions can be monitored for avalanche activity consistently during a winter.
The depositional part of avalanches, so-called avalanche debris is detectable in Sentinel-1 images due to their high surface roughness creating higher backscatter than surrounding undisturbed snow (Eckerstorfer et al., 2017).
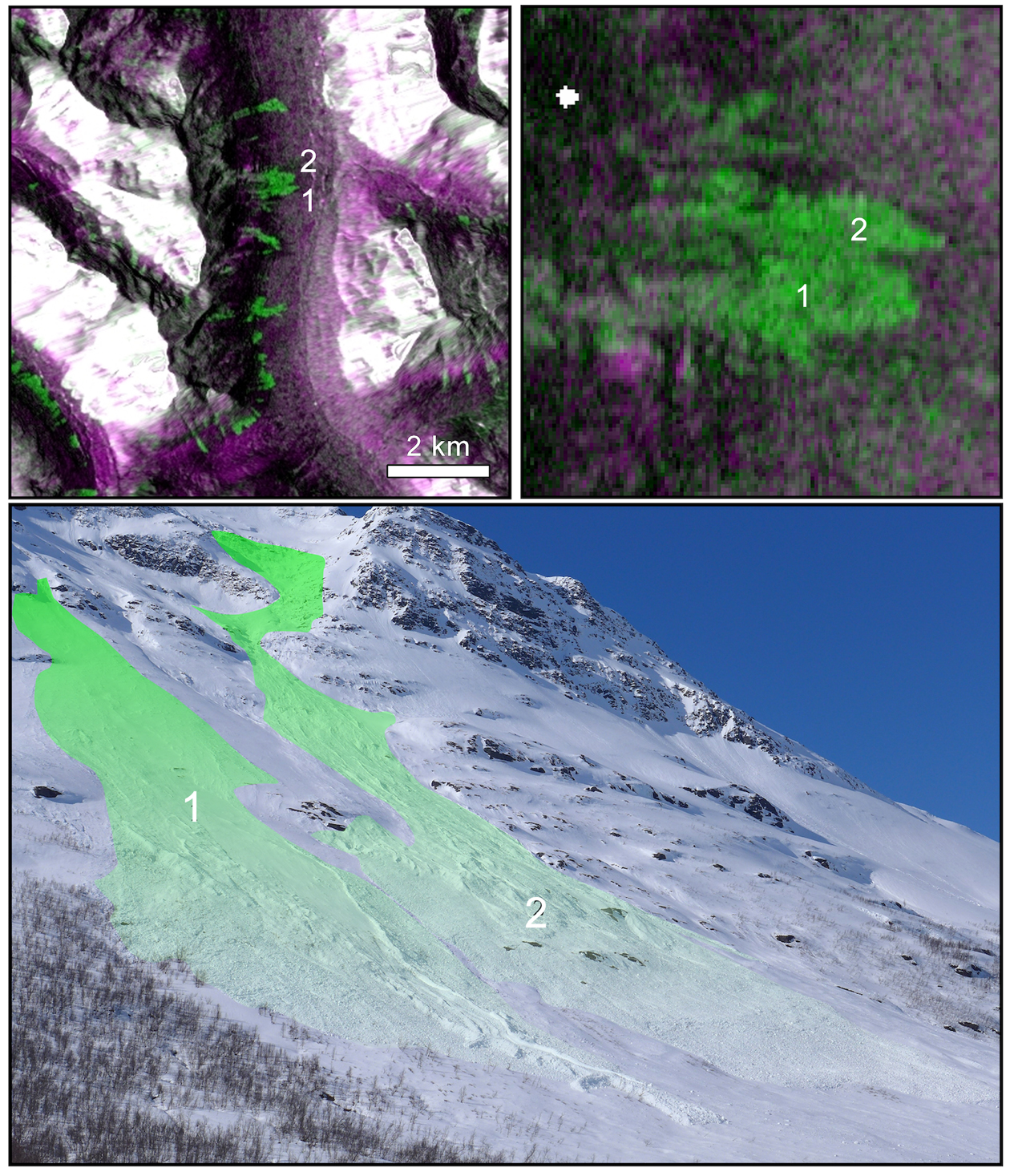
The operational processing chain was developed together with the Norwegian Water and Energy Directorate (NVE) that issues public avalanche forecasts in Norway. The chain is regularly tested in four avalanche forecasting regions in Norway.
NORCE identified the opportunity to demonstrate worldwide avalanche detection in Sentinel-1 images and has been awarded a contract by ESA. In the ongoing ESA open call project, the processing chain is running in six different test regions worldwide (Aosta valley, Davos region, NW-Iceland, East-Afghanistan, Oregon and Colorado).
2016-2018 avalanche activity in Tromsø County, Norway
The extent of the monitored region is roughly 150 x 100 km, covered by four ascending and six descending geometry tracks. This data coverage led to daily coverage with Sentinel-1 images in the winter 2017-2018 with only 1 image missing due to unknown reasons. In fact, on average 7.3 images covered the region within every 6 days repeat cycle in 2017-2018. During the winter 2016-2017, still 26 days were without coverage, which is a significant increase from the first winter Sentinel-1 data became available in 2014-2015 where 140 days during winter (December – May) were missing.
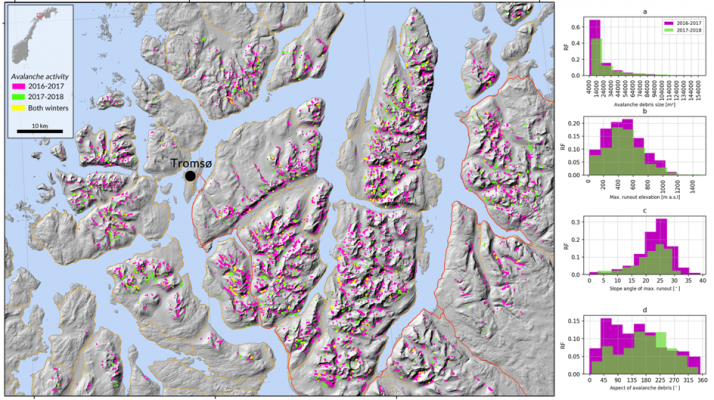
Nearly 80 % more avalanches were detected in winter 2016-2017 than in winter 2017-2018 due to weather conditions being more conducive to triggering avalanches (Figure 2. Left panel). Nevertheless, spatial occurrence of avalanches in both winters was similar, which resulted in nearly 70 % of all avalanches releasing at the same location again. Figure 2 right panel shows histograms of four statistical parameters. 4000 m2 is the minimum cut-off size of detected avalanches. We detected avalanches at sea level as well as in elevations above 1200 m a.s.l with the highest mountains in the region reaching 1800 m a.s.l. The avalanches stopped at slope angles averaging 25 deg, in all slope aspects.
Performance of the automatic avalanche detection
The avalanche detection algorithm developed applies dynamic thresholding of backscatter intensity, difference of gaussian filtering and segmentation to temporal change detection images. Comparing automatic detections to manual interpretations, the algorithm achieves probabilities of detection (PODs) between 82 % and 55.7 %. Corresponding false alarm rates (FARs) range between 26.4 % and 13.8 % (Eckerstorfer et al., 2018). The variation in sensitivity stems from the algorithm’s dependency on snow conditions in the change detection images. In cases of net increase in backscatter in the image due to snow transitioning from dry to wet, PODs are well above 80%. Vice versa, a net decrease in relative backscatter results in small backscatter difference between avalanche debris and surrounding snow and thus low PODs and high FARs.
Comparing the accuracy of the automatic algorithm to in situ observations of avalanches, the achieved POD is 61.6 %. However, the algorithm was capable of detecting all avalanches in the in situ dataset that were large (capable of destroying a house) and 90 % of all medium sized avalanches (capable of burying a car). Nevertheless, only roughly 30 % of all small avalanches (capable of burying a person) were detected.
The use of convolutional neural networks in detecting avalanches in Sentinel-1 images is currently being tested, resulting in an accuracy of consistently over 90 % compared to manual detections (Kummervold et al., 2018).
Worldwide avalanche detection using the Sentinel missions of EU’s Copernicus programme
The Sentinel-1 constellation (S1A and S1B) covers all snow-covered mountain regions worldwide with the exception of the Transantarctic Mountains (Figure 3). Despite the general spatial coverage, revisit frequency and the availability of ascending and descending geometry data is critical. Only in Europe, large parts of the continent are covered by data of both geometries with 6 days repeat frequency. Using overlapping tracks, revisit frequency can be typically 2-3 days in the European Alps and daily coverage at higher latitudes.
Thus, the use of the automatic processing chain to detect avalanches is of operational use in Europe mainly. However, a 12 days revisit frequency allows for consistent monitoring of avalanche activity during entire winters anywhere on Earth, assisting in building long-term datasets that are useful for climate change related studies.
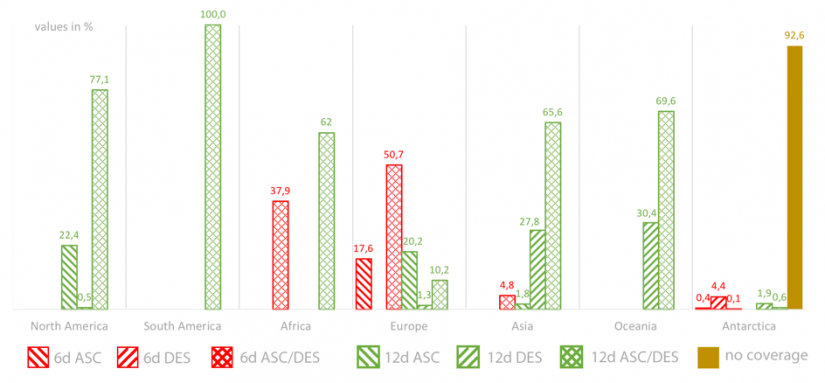
References:
Eckerstorfer, M., Malnes, E., Müller, K., 2017. A complete snow avalanche activity record from a Norwegian forecasting region using Sentinel-1 satellite-radar data. Cold Reg. Sci. Technol. 144, 39–51. http://dx.doi.org/10.1016/j.coldregions.2017.08.004
Eckerstorfer, M., Malnes, E., Vickers, H., Müller, K., Engeset, R., Humstad, T., 2018. Operational avalanche activity monitoring using radar satellites: From Norway to worldwide assistance in avalanche forecasting. Int. Snow Sci. Workshop Proc. 2018 Innsbr. Austria 333–337.
Kummervold, P.E., Malnes, E., Eckerstorfer, M., Arntzen, I.M., Bianchi, F., 2018. Avalanche detection in Sentinel-1 radar images using convolutional neural networks. Int. Snow Sci. Workshop Proc. 2018 Innsbr. Austria 377–381.
Featured image : Avalanche in Tromsø County seen from a drone. Courtesy of Norut