Sen2Cor is the official ESA Sentinel-2 ground segment processor which performs atmospheric correction of Top of Atmosphere images (L1C) to return several outputs, including Surface Reflectance images (L2A) and a Scene Classification (SCL).
The Scene Classification derived with the current version of the Sen2Cor provides pixel-based information on vegetated/non-vegetated areas, water, cloud probabilities, thin cirrus, snow, casted and cloud shadows together with quality information and it is tailored to facilitate the main scope of the L2A processing that is Atmospheric Correction of L1C products.
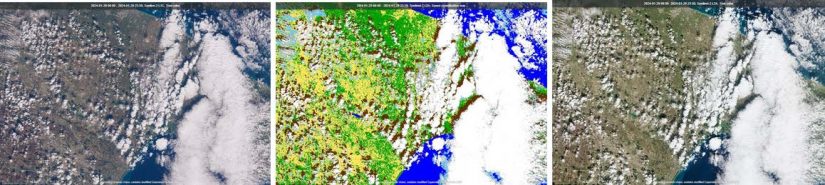
This systematic classification at global scale does not include the detection of artificial objects and the effects of human activities on the observed areas. This ability is becoming more and more crucial for cross-comparing observations over time and determining, for instance, anthropogenic impacts. The Copernicus Sentinel-2 mission data products and Sen2Cor are the best framework to investigate the feasibility of having a systematic classification at global scale also for these additional objects and features.
AI power
AI algorithms applied to remote sensing observations constitute an invaluable resource in environmental monitoring and can help unlocking insights from vast and complex datasets such as those obtained by the Copernicus Sentinel-2 mission, with the first processing baseline delivered in 2015 and its subsequent daily dissemination.
The AI4Sen2Cor project, funded within the FutureEO Permanently Open Call scheme, has been exploring the potential to extend Sen2Cor capabilities with AI-based modules allowing systematic object and feature detection, and monitoring of temporal changes.
State-of-the-art deep learning-based models were trained on a carefully selected and labelled dataset covering vast geographical areas, enabling the training and testing of a high number of variances from a given feature.
Sample detections
Object detections at single and multiple points in time, and statistics associated with multitemporal distribution analysis have been derived, and a few examples are presented in the next figures and graphs.
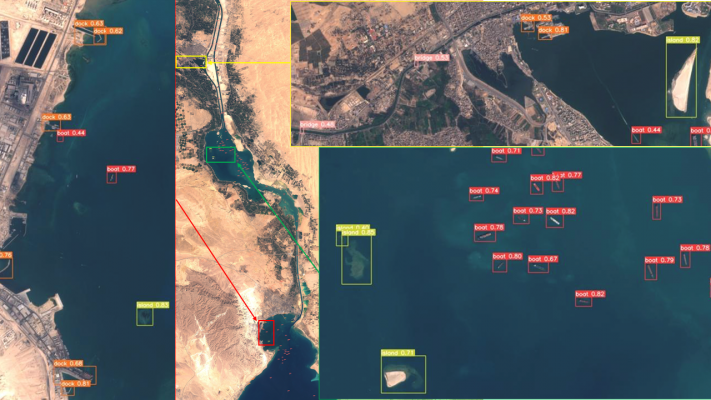
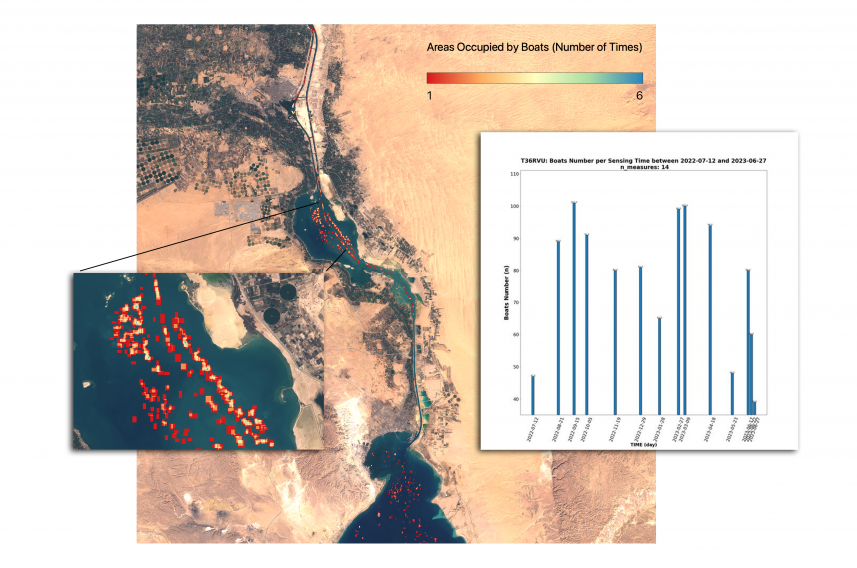
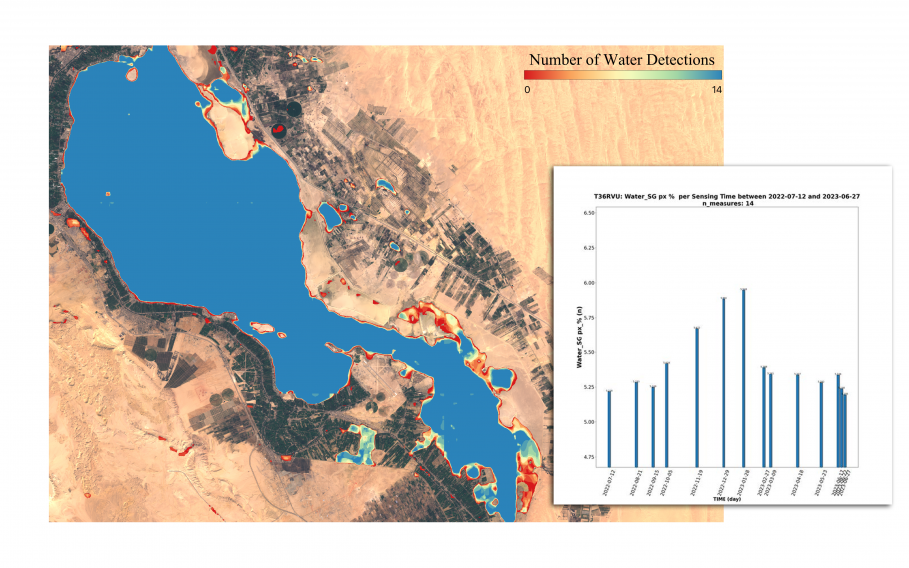
Integration of the new AI tool in the Sen2Cor processor
The developed AI modules have been integrated in Sen2Cor, as displayed in the architecture diagram below, and allow automated feature detection and production of enhanced EO data by leveraging the existing Sen2Cor processor.
All the developed plug-ins and models can be extended by incorporating new inference models, to detect additional features such as energy infrastructures, in the context of the green transition, and changes within the scene in the frame of climate/terrain monitoring and analysis.
On the other hand, future versions of AI4Sen2Cor can be envisioned and tailored to improve and facilitate the processing of L1C products by enhancing the current version of Sen2Cor in operation with AI-based technologies.
As an associated activity, Telespazio Germany is now ingesting the produced training dataset and models into the Earth Observation Training Data Lab (EOTDL), where users will be able to access them and use for their purposes following the EOTDL guidelines.
Telespazio Germany is currently in charge, together with Telespazio France, of the Sen2Cor corrective and perfective maintenance and evolution within the Optical-Mission Performance Cluster (OMPC).